Sanketh Vedula
PhD Candidate, Technion • Pre-doctoral Visiting Scientist, IST Austria
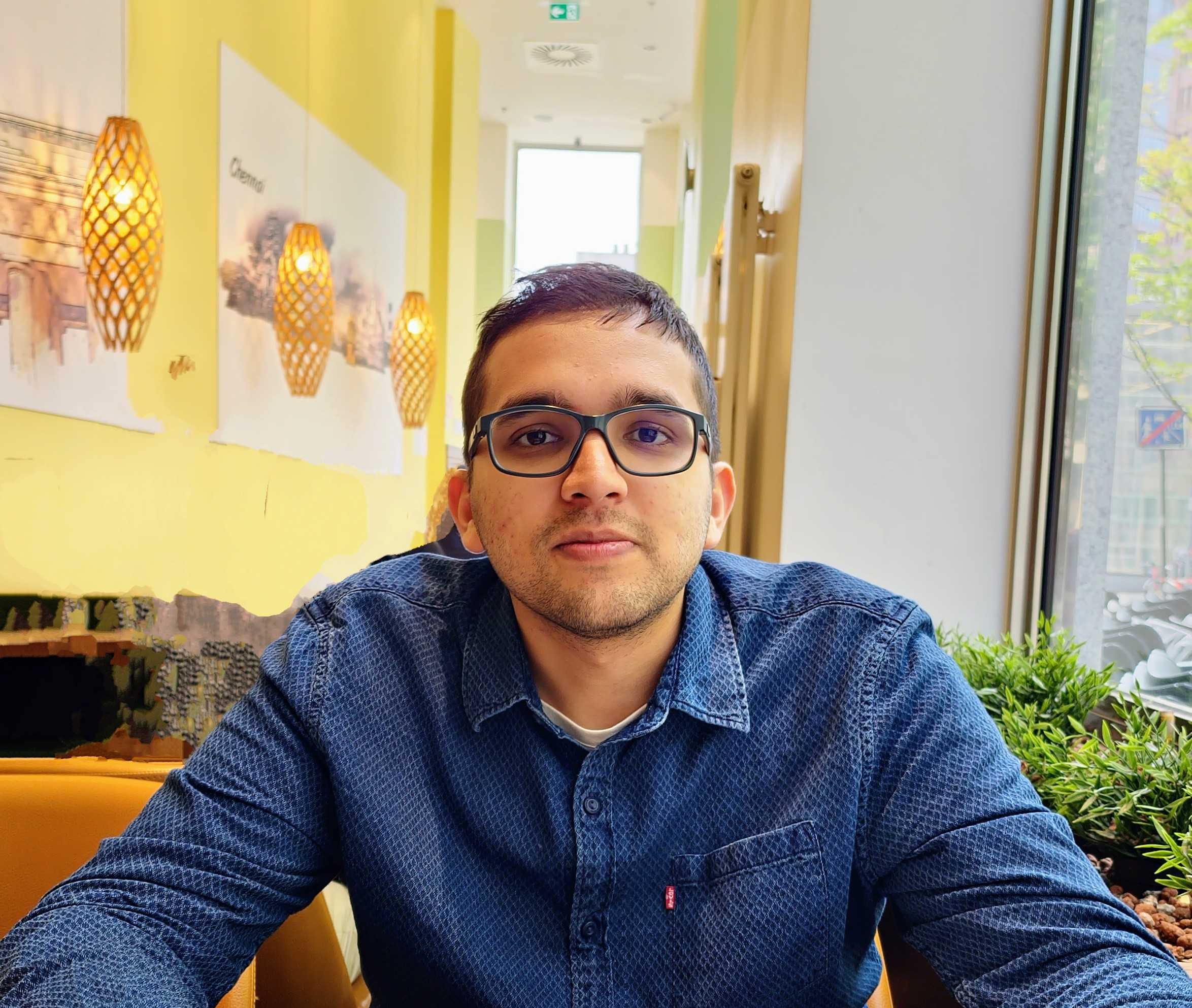
I’m a PhD student at the Computer Science Department, Technion – Israel Institute of Technology advised by Alex Bronstein.
I am interested in developing machine learning methods that accelerate scientific discovery. In particular, I research and develop tools involving generative modeling, optimal transport, geometric deep learning, and distribution-free uncertainty estimation, to tackle problems in computational imaging, computational and structural biology, computational chemistry, and physics.
I aspire to build solutions for challenging problems that have real-world impact. I love science. I enjoy doing research, learning, and discussing ideas with people.
In parallel to my graduate studies, I was an ML Researcher at Sibylla from 2020-23, where I built mid-frequency quant-trading and portfolio management strategies. In 2024, I spent 8 months as an ML Research Scientist intern at Pfizer, Berlin working on problems in pharmaceutical chemistry and structural biology with Djork-Arné Clevert, Kristof Schütt, and Joren Retel.
I received an M.Sc. (cum laude) in Computer Science from Technion in 2020, where I was advised by Alex Bronstein and Michael Zibulevsky. Earlier, I received a B.Eng. (Hons.) in Computer Science from BITS Pilani in 2017. I also held visiting positions at Institute of Science & Technology Austria, Imperial College London, and Chennai Mathematical Institute.
news
Jun 1, 2024 | Preprint on scalable Gromov-Wasserstein solvers |
---|---|
May 1, 2024 | Technion-Pfizer Fellowship |
Jan 14, 2024 | Manifold VQR at AISTATS 2024 |
Jan 1, 2024 | Visiting IST Austria |
Oct 28, 2023 | Awarded excellence scholarship by ISTRC (2023-24) |
Oct 20, 2023 | Two papers accepted at VLDB 2024 |
Jun 19, 2023 | Two papers accepted to ICML Workshops |
Feb 7, 2023 | GRACE at ASPLOS 2023 |
selected publications
- ICML WorkshopScalable unsupervised alignment of general metric and non-metric structuresarXiv preprint arXiv:2406.13507 (AI for Science Workshop, ICML), 2024
- bioRxivSeeing Double: Molecular dynamics simulations reveal the stability of certain alternate protein conformations in crystal structuresbioRxiv, 2024
- ICML WorkshopContinuous Vector Quantile RegressionIn ICML Workshop on New Frontiers in Learning, Control, and Dynamical Systems, 2023
- AISTATSVector Quantile Regression on ManifoldsIn Proc. International Conference on Artificial Intelligence and Statistics (AISTATS), 2024
- MSMLSpectral Geometric Matrix CompletionIn Proceedings of the Second Mathematical and Scientific Machine Learning Conference (MSML), 2021